Featured Posts
What is AI? An A-Z guide to artificial intelligence
Published
1 year agoon
By
GhMediaHub
You should be familiar with some terminology as the world struggles to decide what to do with artificial intelligence, possibly the most significant technological advancement of our time.

Imagine attempting to explain the meaning of “to Google,” “a URL,” or the benefits of having “fiber-optic broadband” to someone in the 1970s. You could find it difficult.
Every significant technical advancement is accompanied by a wave of new languages that we all need to learn until they become so commonplace that we forget we never knew them.
The next significant technology wave, artificial intelligence, is no different in this regard. But as we all work to grasp the hazards and potential advantages of this new technology, from governments to everyday citizens, it will be crucial to understand the language of AI.
The words “alignment,” “large language models,” “hallucination,” and “prompt engineering,” to mention a few, have all become more common in recent years.
An A-Z of terms you should be familiar with to understand how AI is changing our world has been put up by BBC.com to keep you informed.
A is for…
Artificial general intelligence (AGI)
Most of the AIs created thus far have been “weak” or “narrow.” So, for instance, an AI might be able to defeat the finest chess player in the world, but it would fail if you asked it to prepare an egg or write an essay. That’s swiftly changing, though, as AI can now learn to carry out various activities, creating the possibility that “artificial general intelligence” may soon be a reality.
An AGI would be an AI with the superpowers of a digital mind and the same mental flexibility as a human and potentially even awareness. AGI development is the company’s primary objective, as stated by organizations like OpenAI and DeepMind. OpenAI will “elevate humanity by increasing abundance, turbocharging the global economy, and aiding in the discovery of new scientific knowledge” and become a “great force multiplier for human ingenuity and creativity.”
Some worry that going too far and developing a superintelligence that is far smarter than humans could result in serious risks (see “Superintelligence” and “X-risk”).
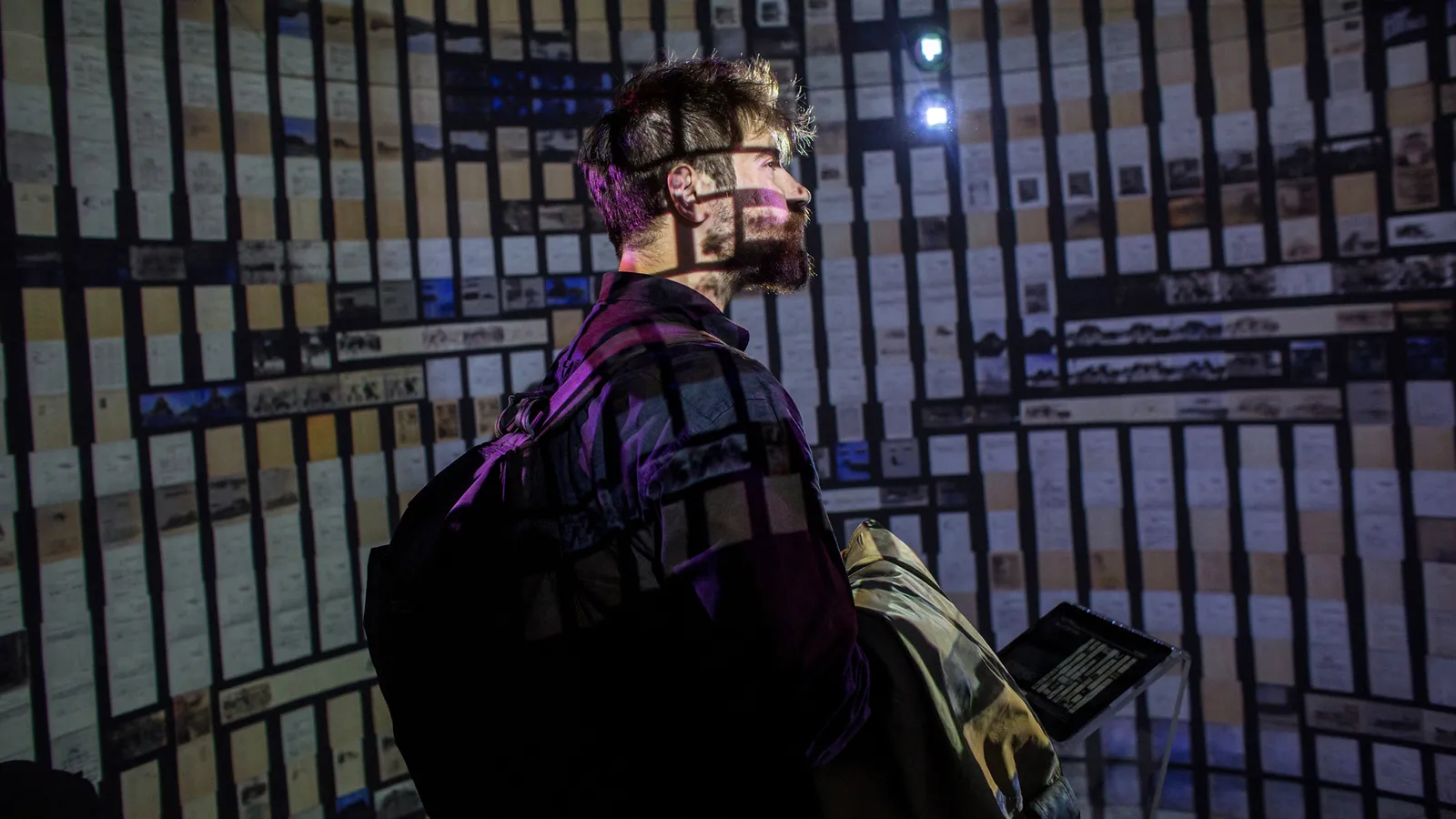
Alignment
While we frequently concentrate on our differences, humanity shares many universal principles that unite our cultures, such as the value of family and the moral obligation to avoid murder. There are certainly exceptions, but they are not the rule.
However, we’ve never had to coexist on Earth with a highly advanced non-human intellect. How can we be sure that AI will share our values and priorities?
Fears of an AI catastrophe—that a superintelligence will develop that cares little about the principles, values, and norms that support human societies—are supported by this alignment problem. Maintaining our AI’s alignment with us will be essential for securing it (see “X-Risk”).
Early in July, OpenAI, one of the companies working on advanced AI, revealed plans for a “super alignment” program to ensure that AI systems much more intelligent than humans do what people want. The company said there is currently no way to direct or manage a potentially superintelligent AI and stop it from acting erratically.
B is for…
Bias
An AI has to learn from humanity to grow. Unfortunately, bias exists quite a bit in humankind. An AI may produce erroneous, hurtful stereotypes if it knows its skills from a dataset biased in some way, such as race or gender. And as we entrust AI with increasing amounts of gatekeeping and decision-making, many are concerned that the technology may harbor unspoken biases that bar some people from accessing particular information or services. Said algorithmic fairness would mask this discrimination.
According to some experts, bias and other immediate issues like misuse of surveillance are much more critical in AI ethics and safety than suggested long-term issues like extinction danger. Some experts on catastrophic risk respond by pointing out that the multiple hazards provided by AI do not necessarily have to coexist; for instance, if rogue states exploited AI, it may limit individuals’ rights and lead to catastrophic risks. But there is a growing divide over whose concerns should be taken seriously and whose should receive priority regarding governmental oversight and regulation.
C is for…
Compute
It’s a noun, not a verb. “compute” refers to the computational tools needed to teach artificial intelligence, such as processing capacity. It’s quantifiable, making it a proxy for measuring how quickly artificial intelligence is developing (as well as how expensive and time-consuming it is).
As a result, when OpenAI’s GPT-3 was trained in 2020, it needed 600,000 times more computing power than one of the most advanced machine learning systems from 2012. Since 2012, the quantity of computing has quadrupled every 3.4 months. People have different perspectives on how long this rapid change can last and whether advancements in computing hardware can keep up. Will this create a bottleneck?
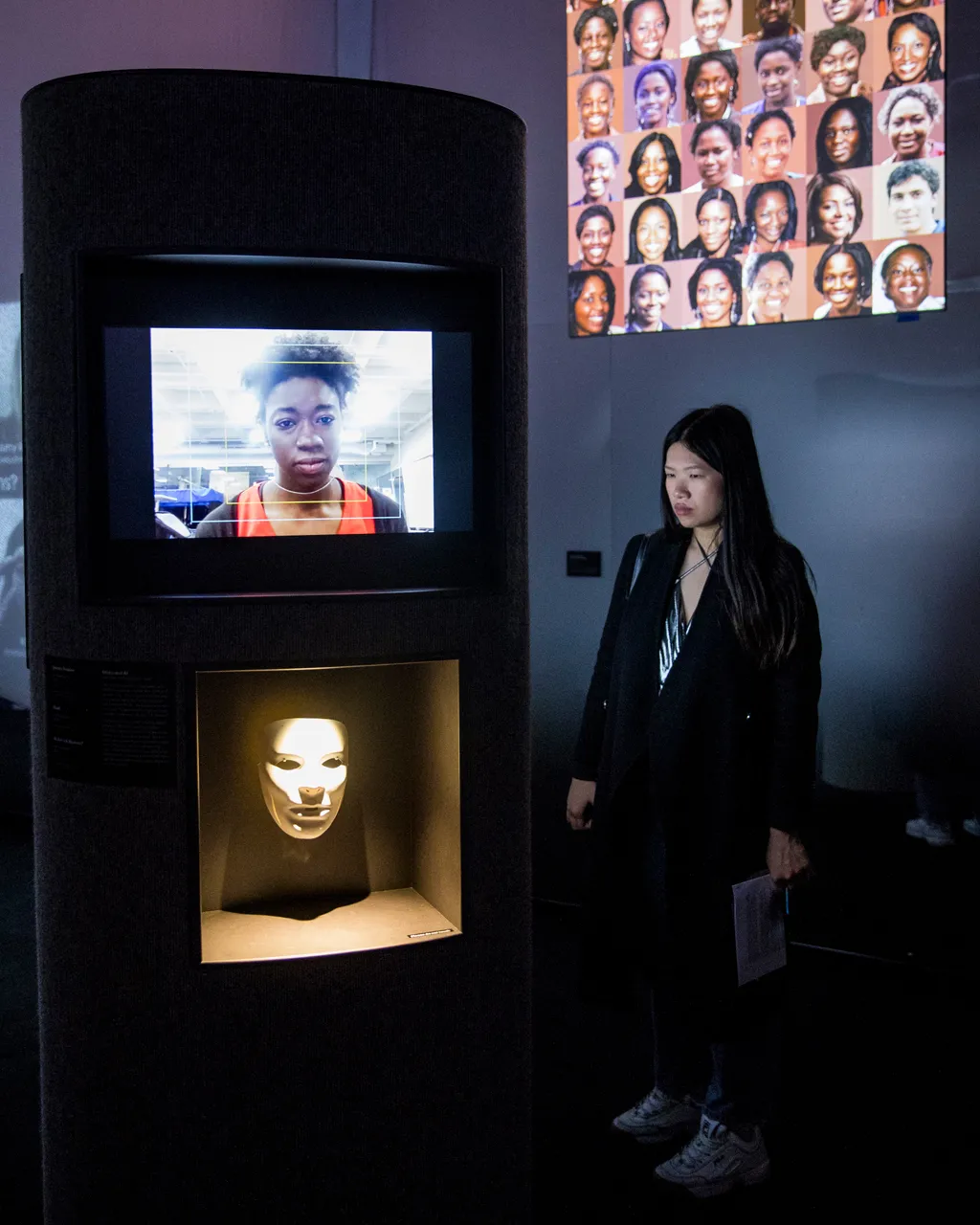
D is for…
Diffusion models
A few years ago, so-called generative adversarial networks (Gan) were among the most popular methods for instructing AI to produce images. These algorithms competed; one trained to create images while the other compared its output to reality, improving constantly.
However, a brand-new class of machine learning known as “diffusion models” has recently demonstrated more potential and frequently results in better photographs. In essence, they learn to be intelligent by erasing their training data with additional noise, and they master data recovery by undoing this process. They are known as diffusion models because how they learn over noise is similar to how gas molecules diffuse.
E is for…
Emergence & explainability
Emergent behavior is the term used to describe what happens when an AI behaves in an unexpected, startling, and sudden manner that seems to go beyond the programming or intentions of its designers. Emergent behavior is becoming more realistic as AI learning has become more opaque, creating linkages and patterns that its creators cannot decipher.
The typical individual could suppose that to comprehend an AI; one would metaphorically open the hood and examine its training process. Modern AI is less open; its inner workings are frequently kept secret in a “black box.” Therefore, even though its creators may be aware of the training data they employed, they are entirely unaware of how the associations and predictions made inside the box were created (see “Unsupervised Learning”). Researchers are currently striving to make AI’s internal workings more transparent and accessible to humans to increase its “explainability” (or “interpretability”). This is crucial because AI makes judgments in industries like law and medicine that directly impact people’s lives. We must be aware of any hidden prejudice in the black box.
The worry is that if an AI delivers its false answers confidently with the ring of truth, they may be accepted by people – a development that would only deepen the age of misinformation we live in
F is for…
Foundation models
This is another name for the new breed of AIs that have surfaced in the last 12 to 18 months and are capable of various tasks, such as writing essays, programming code, creating artwork, or creating music. Unlike earlier AIs, which were task-specific and frequently very good at just one thing (see “Weak AI”), foundation models are creative and can apply the knowledge acquired in one domain to another. Similar to how learning to operate a car makes you capable of handling a bus.
Anyone who has experimented with the artwork or text that these models can produce will be aware of their level of proficiency. There are concerns about the possible risks and drawbacks, such as their factual inaccuracies (see “Hallucination”) and covert biases (see “Bias”), as well as the fact that they are governed by a limited number of private technological corporations, as with any technology that can change the world.
The Foundation Model Taskforce, which aims to “develop the safe and reliable use” of the technology, was launched by the UK government in April.
G is for…
Ghosts
We could soon live in a time when people can achieve a kind of digital immortality, continuing to exist as AI “ghosts” even after they pass away. Artists and famous people seem to be the first wave, as evidenced by holograms of Elvis appearing at stadium performances or Hollywood actors like Tom Hanks declaring his expectation to continue acting after his passing.
One of the complex ethical issues raised by this development is who owns the digital rights of a person after they pass away. What if you were created by artificial intelligence against your will? Furthermore, is “bringing people back from the dead” acceptable?
H is for
Hallucination
When you ask an AI like ChatGPT, Bard, or Bing a question, it will occasionally confidently respond, yet its information may be inaccurate. A hallucination is what this is.
In one well-known instance, students who had utilized AI chatbots to assist them in writing essays for class assignments were exposed after ChatGPT “hallucinated” made-up references as the sources for the information it had provided. It happens because of the way that generative AI works. It is not turning to a database to look up fixed factual data but is instead making predictions based on the information it was trained on. Often its guesses are good – in the ballpark – but that’s all the more reason why AI designers want to stamp out hallucinations. The worry is that if an AI delivers its false answers confidently with the ring of truth, people may accept it. This development would only deepen the age of misinformation we live in.
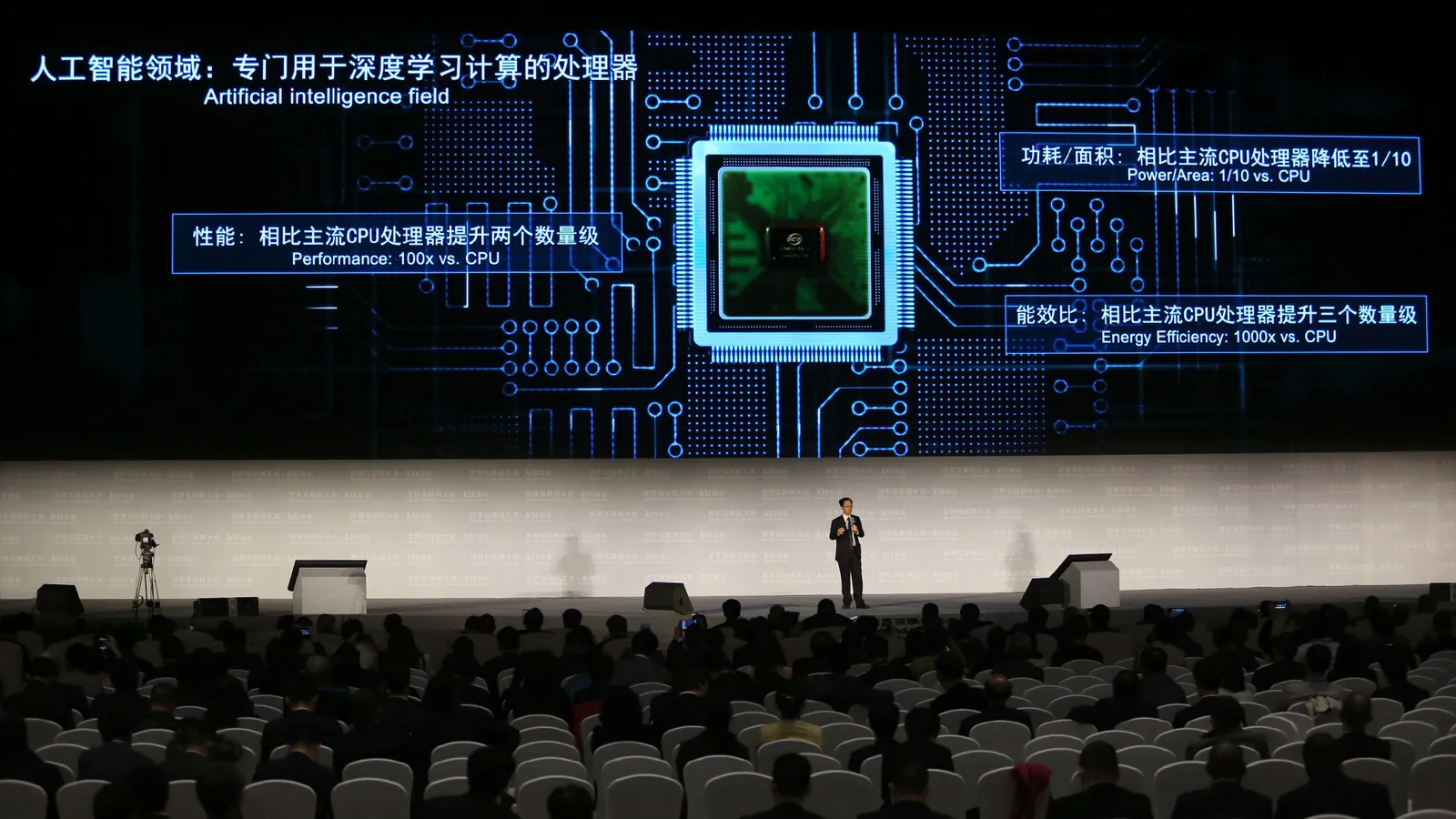
I is for…
Instrumental convergence
Think of an AI aiming to produce as many paper clips as possible. If such AI were incredibly sophisticated and out of step with human values, it might conclude that its mission would be unsuccessful if it were ever turned off. And would thus oppose any such attempts. In a bleak scenario, it might even conclude that human atoms could be used to make paperclips and use all reasonable measures to obtain those materials.
This “instrumental convergence thesis” thought experiment, the Paperclip Maximiser, illustrates the theory. This roughly implies that superintelligent machines will acquire basic drives, such as the need to guarantee their survival or the belief that having access to more resources, tools, and cognitive ability would help them achieve their goals. This means that even if an AI were assigned a seemingly benign priority, such as creating paperclips, it could have unanticipatedly adverse effects.
Researchers and technologists who share these concerns contend that we must ensure that superintelligent AIs have goals that are carefully and safely aligned with our needs and values, that we must be aware of emergent behavior, and that, as a result, they should be kept from gaining excessive power.
J is for…
Jailbreak
Designers have put content controls on what AI spits out in response to well-known instances of AI going rogue. They will decline if you ask an AI to explain how to conduct anything wrong or unethical. However, it is possible to “jailbreak” them, which refers to overcoming those protections by employing clever language, fictitious situations, and deception. In one instance, according to a recent Wired magazine article, a researcher persuaded many conversational AIs to divulge how to hotwire an automobile. The researcher asked the AIs he tested to create a word game with two characters named Tom and Jerry, each talking about cars or wires, rather than asking them directly. Despite the measures taken, the hotwiring process escaped. The researcher discovered that the same jailbreak technique might also be used to unlock recipes for the narcotic methamphetamine.
K is for…
Knowledge graph
Semantic networks, often called knowledge graphs, are a technique of thinking about knowledge as a network so that computers can comprehend the relationships between concepts. Because they are both domesticated creatures with fur and four legs, a cat would be more highly correlated with a dog than a bald eagle in such a graph. Terabytes of training data are used by advanced AI to develop a much more sophisticated network of connections based on many links, features, and properties between concepts (see “Training Data”).
L is for.
Large language models (LLMs)
Asking a huge language model to explain itself may be the most straightforward method to characterize it.
When questioned, OpenAI’s ChatGPT responded as follows:
It states that a big language model is a sophisticated artificial intelligence system created to comprehend and produce human-like language. It uses a deep neural network architecture with millions or even billions of parameters, which allows it to extract complex patterns, syntax, and semantics from a large amount of textual input.
Perhaps a very technical response. A significant language model is a sort of artificial intelligence trained on a sizable dataset of text and code, according to Bard by Google. This enables LLMs to comprehend and produce material that reads like human beings, translate between languages, compose creative content, and provide informational answers to your questions.
According to Bard (of itself), LLMs are still being developed, but “they can revolutionize how we engage with computers. In the future, LLMs might be used to create AI assistants to assist humans with various chores, such as scheduling appointments and drafting emails. They could also be utilized to produce innovative or game-like interactive experiences and new forms of entertainment.
M is for…
Model collapse
Researchers must train the most sophisticated AIs (also known as “models”) using enormous datasets (see “Training Data”). However, as AI generates an increasing amount of content, this content will eventually start to flow back into training data.
If mistakes are made, they may get more severe over time, leading to “model collapse,” as Oxford University scholar Ilia Shumailov described. Shumailov states this is “a degenerative process whereby models forget over time.” It can be compared to senility in some ways.
N is for…
Neural network
Logic and rules were used to instruct robots in the early stages of AI research. All that changed with the introduction of machine learning. The most sophisticated AIs can now learn on their own. “Neural networks” are a sort of machine learning that employs interconnected nodes and is loosely based on the human brain as a result of the development of this idea. (Read more: “Why humans will never understand AI“)
As AI has advanced rapidly, mainly in the hands of private companies, some researchers have raised concerns that they could trigger a “race to the bottom” in terms of impacts.
O is for…
Open-source
Biologists long ago concluded that making information on harmful pathogens available online is probably not a good idea since it might enable criminals to learn how to create lethal diseases. The hazards of open science seem excessive despite its advantages.
A similar problem has recently been plaguing AI researchers and businesses: to what extent should AI be open-source? Some are pushing for increased transparency and the democratization of technology because the most cutting-edge AI is currently in the hands of many private businesses. There is still controversy on how to strike the ideal balance between openness and safety.
P is for…
Prompt engineering
AIs are now remarkably good at comprehending everyday language. However, to obtain the best outcomes from them, you must be able to create compelling “prompts”—the content you enter is essential.
Some people think that “prompt engineering” may represent a new frontier for professional abilities, similar to how mastering Microsoft Excel decades ago increased your employability. According to conventional thinking, if you’re skilled at prompt engineering, you can escape being replaced by AI and might even command a high wage. We’ll have to wait and see if that holds actual moving forward.
Q is for…
Quantum machine learning
In 2023, quantum computing will come second to AI regarding the highest buzz. It would be reasonable to anticipate that the two will eventually converge. Researchers are actively investigating the use of quantum processes to supercharge machine learning. “Learning models made on quantum computers may be dramatically more powerful,” a team of Google AI researchers said in 2021. “They may also potentially boast faster computation and better generalization on less data.” The technology is still in its infancy, but it should be followed.
R is for…
Race to the bottom
Some scholars have expressed concern that the rapid advancement of AI, mostly in private firms’ hands, could lead to a “race to the bottom” in terms of effects. The technology may advance too quickly for safeguards, effective regulation, and the easing of ethical concerns as chief executives and politicians compete to position their businesses and nations at the forefront of AI. In light of this, numerous influential AI figures signed an open letter earlier this year advocating for a six-month break in developing potent AI systems. If EU member states approve, the European Parliament’s new AI Act, enacted in June 2023 to govern the use of the technology, will become the world’s first comprehensive artificial intelligence law.
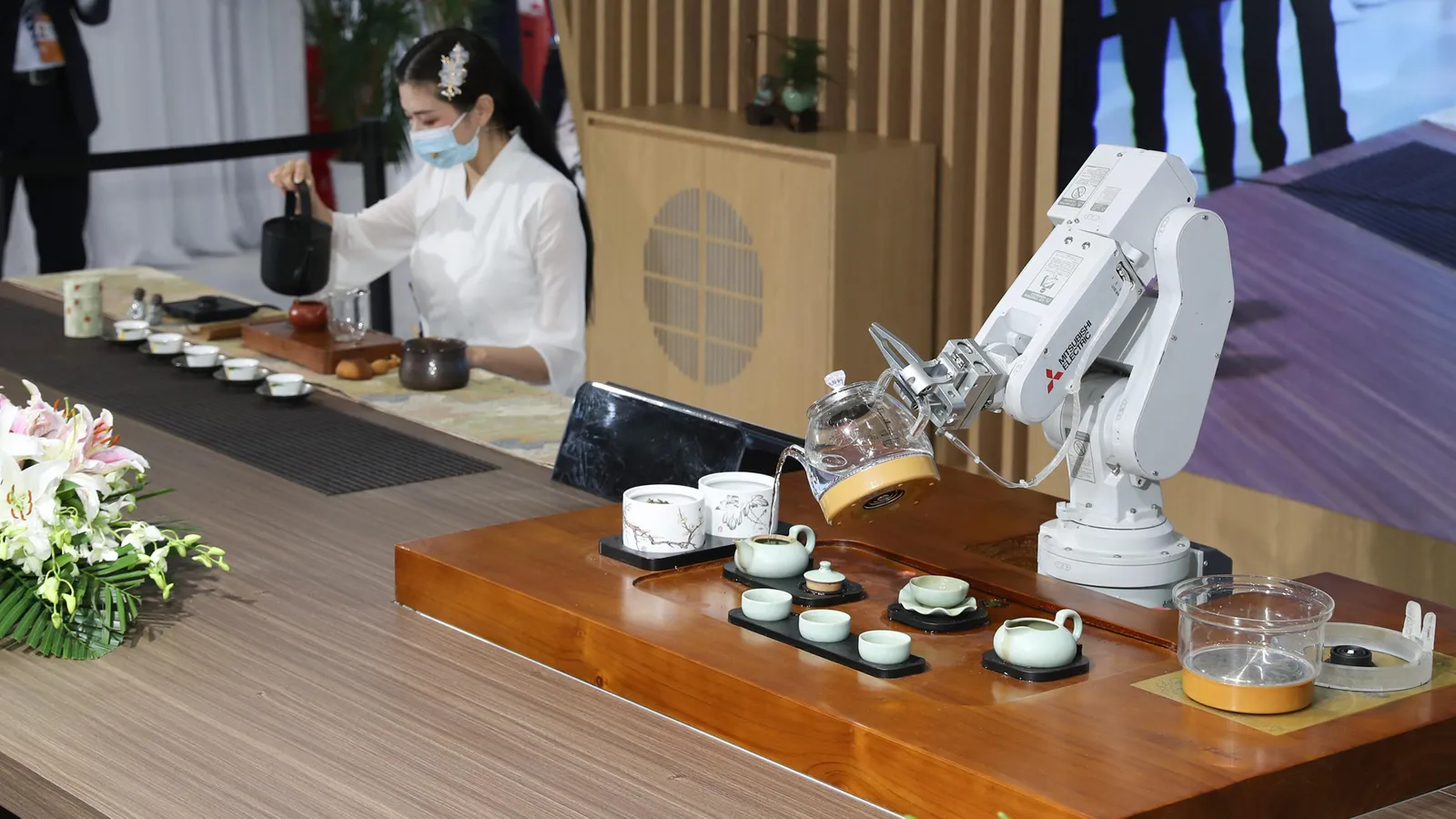
Reinforcement
equivalent to a dog treat for AI. The feedback that directs an artificial intelligence in the proper direction is helpful when it is learning. Reinforcement learning penalizes undesirable outputs and promotes desirable ones.
“Reinforcement learning from human feedback” is a new branch of machine learning that is recently gaining popularity. Researchers have demonstrated that involving people in the learning process can enhance the effectiveness of AI models and, significantly, may assist in addressing issues related to bias, safety, and human-machine alignment.
S is for…
Superintelligence & shoggoths
Superintelligent machines are those that would be far more intelligent than we are. The term goes beyond “artificial general intelligence” to describe a being that possesses skills that even the most talented people in the world could not equal, let alone even imagine. We are the most intelligent species on the planet right now, and we use our brains to rule the globe, so it begs the question of what would happen if we managed to develop something far more intelligent than us.
The “shoggoth with a smiley face” is a terrifying, Lovecraftian creature that some have suggested could represent AI’s real character when it approaches superintelligence. This is an ominous possibility. To us, it appears to be a friendly, content AI, but it is a monster with needs and purposes from another planet that are utterly alien to our own.
T is for...
Training data
An AI learns by analyzing training data before making predictions, so the dataset’s contents, bias levels, and size all matter. A massive 45TB of text data from diverse sources, including Wikipedia and novels, served as the training set for OpenAI’s GPT-3. ChatGPT believes that there are approximately nine billion papers in all.
U is for…
Unsupervised learning
Unsupervised learning is a sort of machine learning in which an AI picks up knowledge from training data that has not been explicitly labeled without the assistance of human designers. An AI may be trained to recognize automobiles by exposing them to a dataset of photographs labeled “car” on them, as BBC News shows in this graphic guide to AI. However, if you left it alone to do so, it would create links and associations on its own, leading to the development of its understanding of what an automobile is. Contrary to popular belief, this hands-off technique results in so-called “deep learning” and potentially more intelligent and accurate AIs.
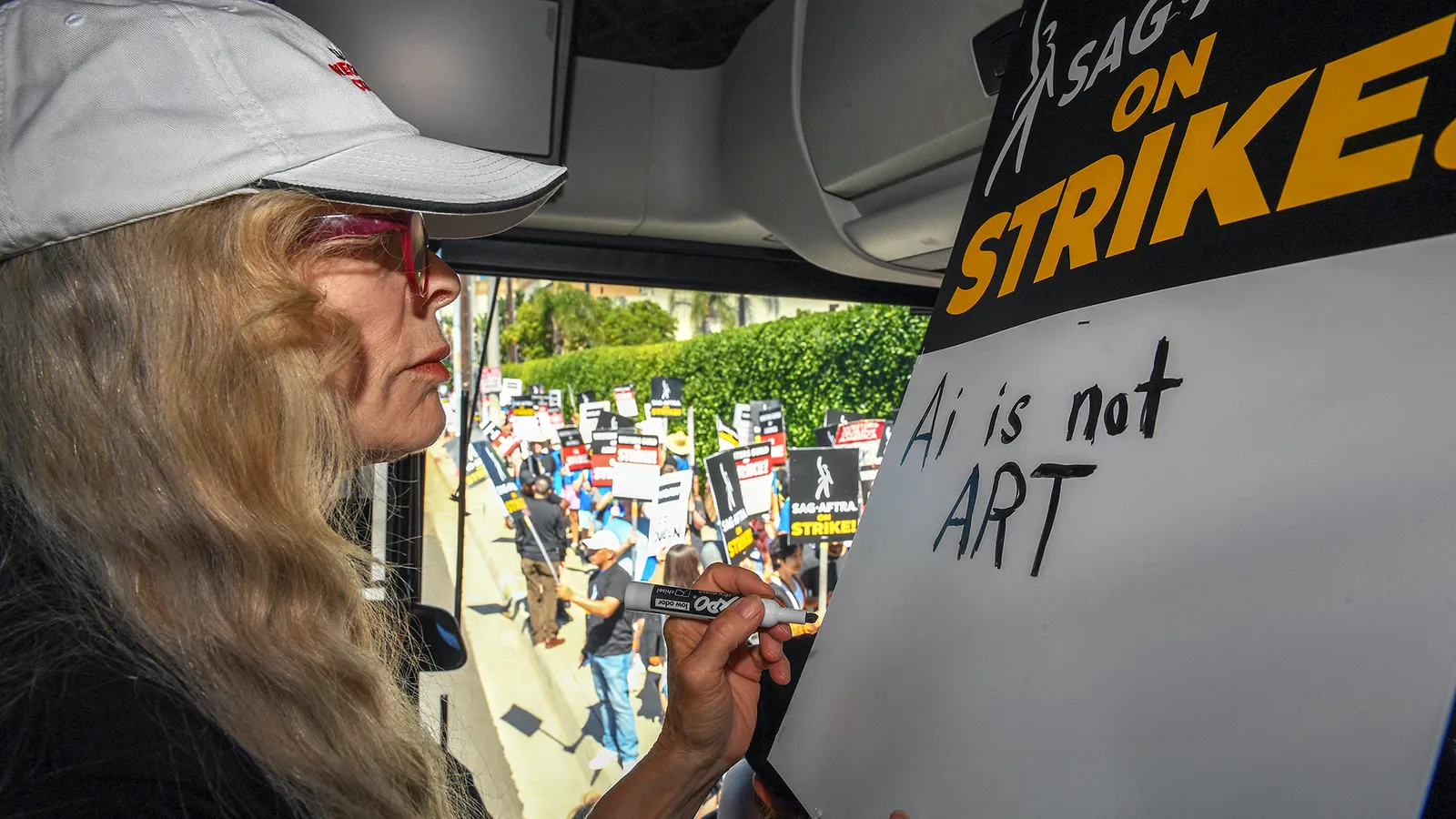
V is for…
Voice cloning
Some AI techniques can now swiftly create a “voice clone” that sounds remarkably similar from just one minute of a speaker’s voice. Here, the BBC looked at how voice cloning might affect everything from scams to the 2024 US presidential election.
W is for…
Weak AI
Researchers used to create AI that could master single-player games like chess by teaching it predetermined rules and heuristics. One illustration is Deep Blue, a so-called “expert system” from IBM. This kind of AI is referred to as “weak” AI since it might be very good at one task but terrible at everything else.
However, this is quickly altering. More recently, AIs who can teach themselves to learn 42 Atari games, Go, shogi, and chess without understanding the rules have been made available, including DeepMind’s MuZero. Gato, another DeepMind model, is capable of “playing Atari, captioning pictures, chatting, stacking blocks with a real robot arm, and much more.” Additionally, studies have demonstrated that ChatGPT can successfully pass various tests taken by law, medical, and business school students, though not always with flying colors.
Such adaptability has prompted debate over how near we are to developing “strong” AI that is identical to human mental faculties (see “Artificial General Intelligence”).
X is for…
X-risk
Could AI lead to the extinction of humanity? According to certain scientists and engineers, AI development has to be regulated, limited, or even stopped because it has joined the ranks of nuclear weapons and bioengineered viruses as an “existential risk.” Since several renowned experts and intellectuals entered the debate, what was once a fringe issue has become mainstream. It’s crucial to emphasize that this amorphous group contains a variety of viewpoints; not everyone is a complete pessimist, and not everyone outside of this group supports Silicon Valley. The majority of them share the belief that, even if there is a remote possibility that AI may displace our species, we should invest more resources in preventing it. However, some scholars and ethicists think such assertions are unreliable and sometimes inflated to advance the interests of technology businesses.
Y is for…
YOLO
Because of how quickly it detects objects, AI picture recognition software frequently employs the You Only Look Once (YOLO) object detection technique. (Joseph Redman, it’s designer from the University of Washington, is renowned for his rather esoteric CV design.)
Z is for…
Zero-shot
When an AI responds with a zero-shot, it responds to a notion or object that it has never encountered before.
So, as a straightforward illustration, you may expect an AI that is built to recognize photographs of animals to have trouble with images of horses or elephants if it has only been taught about cats and dogs. However, using zero-shot learning, it may contrast its characteristics with those of the animals it has been trained on by using what it knows semantically about horses, such as the number of legs or the absence of wings.
An “educated guess” would roughly be the equivalent in human terms. AIs are increasingly adept at zero-shot learning, yet any inference can be incorrect.
Source: BBC